The problem with traditional technical analysis is that most financial time series (such as stock prices) are pretty close to being a "random walk". The "random walk hypothesis" states that stock market prices evolve according to a random walk and thus cannot be predicted. (This is consistent with the "efficient market hypothesis.") However, stock prices are not a pure random walk. For one thing, there is usually a small deterministic (predictable) component. The implication here is that much of technical analysis is an effort to detect a small deterministic trend within a larger random process. This large random component means that conventional technical analysis often doesn't work all that well.
There is some evidence that certain asset classes, such as currencies and commodities, tend to be mean-reverting, in which case I would expect traditional technical analysis to work somewhat better for these assets. Even so, there is a large random component to these asset prices that make them difficult to forecast. To address the randomness problem, some quantitative analysts use statistical arbitrage, in particular, cointegration. Cointegration involves assembling a portfolio of securities that has favorable statistical properties for forecasting. The idea is that you try to find a combination of 2 or more securities in which the random walk components largely cancel out, and you’re left with a portfolio that is more deterministic.
The most common example of this is pair trading. Pair trading is a market-neutral trading strategy that monitors the prices of two correlated securities, such as, say, Coca-Cola (KO) and Pepsi (PEP). Because these companies sell similar products, their stocks tend to move together. In pair trading you buy one and short the other (in the correct ratio) when their prices diverge significantly (based on historical norms). However, the problem with pair trading is that in recent years it has become too popular. As we know, when too many people are using the same or similar strategy, its effectiveness diminishes. To put it bluntly, it appears that pair trading is dead.
However, there’s no reason to limit cointegration to two securities. Statistical methods such as the Johansen procedure allow one to find cointegrated portfolios of 3 or more securities. The rapid growth in the number of ETFs in recent years adds a large number of potential securities with which to assemble a cointegrated portfolio. The possible number of cointegrated portfolios of 3 or more ETFs is so large that it is unlikely to be fully exhausted for the foreseeable future. (For example, I calculate 1.2 billion possible combinations of 3 ETFs. Even if only a small fraction of these exhibit cointegration, that is still likely to be a large number. The number of possible combinations of 4 or 5 ETFs is astronomical.)
There are commercial software packages (such as FX AlgoTrader) which enable users to find and trade cointegrated pairs, but I’m not aware of any commercial products that enable users to assemble cointegrated portfolios of 3 or more securities. For anyone who has software programming skills, there are resources available to do this. (For example, Ernie Chan’s “Algorithmic Trading: Winning Strategies and Their Rationale” provides a good overview, is practical and not overly technical.) As a software engineer, I wrote my own software to find and trade cointegrated portfolios of multiple (3 or more) securities. Currently, I’m trading a cointegrated portfolio of 3 ETFs, and so far, I’m having good results. I did paper trading for nearly a year while I developed and refined the algorithm, but I recently “went live”. Backtesting suggests I should be able to achieve greater than 50% average yearly return (AYR), with a maximum drawdown of 13%. Since I starting live trading in a $100,000 account 2 1/2 months ago I’ve made an 11.3% return during this period. (If the algorithm continues to perform this well, this suggests an AYR of 67%.) Time will tell how well the algorithm continues to perform.
To implement a mean reversion strategy, I apply a Kalman filter to the cointegrated portfolio. This provides optimal dynamic updating of the portfolio weights, along with error and variances of the “spread”. (This is similar to a Bollinger Band approach, but without the lag inherent in moving averages and moving standard deviations.) The following chart shows 5 years of weekly data of the spread “error” (blue line), along with +/- the square-root of the spread variance (green/red lines). When the spread error is above the green line, I short the portfolio. When it’s below the red line, I buy:
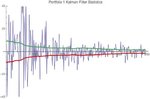
The cumulative returns during 4 years of backtesting produced an in-sample AYR of 67%:
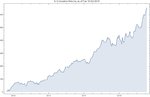
If this thread generates any interest, I’ll try to post regularly to report on the algorithm performance, answer questions, and discuss other trading strategies I’m looking at, such as using multivariate auto-regressive models.
P.S. If anyone can tell me how to make the chart images larger, I'd appreciate it!
There is some evidence that certain asset classes, such as currencies and commodities, tend to be mean-reverting, in which case I would expect traditional technical analysis to work somewhat better for these assets. Even so, there is a large random component to these asset prices that make them difficult to forecast. To address the randomness problem, some quantitative analysts use statistical arbitrage, in particular, cointegration. Cointegration involves assembling a portfolio of securities that has favorable statistical properties for forecasting. The idea is that you try to find a combination of 2 or more securities in which the random walk components largely cancel out, and you’re left with a portfolio that is more deterministic.
The most common example of this is pair trading. Pair trading is a market-neutral trading strategy that monitors the prices of two correlated securities, such as, say, Coca-Cola (KO) and Pepsi (PEP). Because these companies sell similar products, their stocks tend to move together. In pair trading you buy one and short the other (in the correct ratio) when their prices diverge significantly (based on historical norms). However, the problem with pair trading is that in recent years it has become too popular. As we know, when too many people are using the same or similar strategy, its effectiveness diminishes. To put it bluntly, it appears that pair trading is dead.
However, there’s no reason to limit cointegration to two securities. Statistical methods such as the Johansen procedure allow one to find cointegrated portfolios of 3 or more securities. The rapid growth in the number of ETFs in recent years adds a large number of potential securities with which to assemble a cointegrated portfolio. The possible number of cointegrated portfolios of 3 or more ETFs is so large that it is unlikely to be fully exhausted for the foreseeable future. (For example, I calculate 1.2 billion possible combinations of 3 ETFs. Even if only a small fraction of these exhibit cointegration, that is still likely to be a large number. The number of possible combinations of 4 or 5 ETFs is astronomical.)
There are commercial software packages (such as FX AlgoTrader) which enable users to find and trade cointegrated pairs, but I’m not aware of any commercial products that enable users to assemble cointegrated portfolios of 3 or more securities. For anyone who has software programming skills, there are resources available to do this. (For example, Ernie Chan’s “Algorithmic Trading: Winning Strategies and Their Rationale” provides a good overview, is practical and not overly technical.) As a software engineer, I wrote my own software to find and trade cointegrated portfolios of multiple (3 or more) securities. Currently, I’m trading a cointegrated portfolio of 3 ETFs, and so far, I’m having good results. I did paper trading for nearly a year while I developed and refined the algorithm, but I recently “went live”. Backtesting suggests I should be able to achieve greater than 50% average yearly return (AYR), with a maximum drawdown of 13%. Since I starting live trading in a $100,000 account 2 1/2 months ago I’ve made an 11.3% return during this period. (If the algorithm continues to perform this well, this suggests an AYR of 67%.) Time will tell how well the algorithm continues to perform.
To implement a mean reversion strategy, I apply a Kalman filter to the cointegrated portfolio. This provides optimal dynamic updating of the portfolio weights, along with error and variances of the “spread”. (This is similar to a Bollinger Band approach, but without the lag inherent in moving averages and moving standard deviations.) The following chart shows 5 years of weekly data of the spread “error” (blue line), along with +/- the square-root of the spread variance (green/red lines). When the spread error is above the green line, I short the portfolio. When it’s below the red line, I buy:
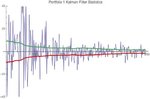
The cumulative returns during 4 years of backtesting produced an in-sample AYR of 67%:
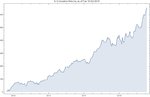
If this thread generates any interest, I’ll try to post regularly to report on the algorithm performance, answer questions, and discuss other trading strategies I’m looking at, such as using multivariate auto-regressive models.
P.S. If anyone can tell me how to make the chart images larger, I'd appreciate it!